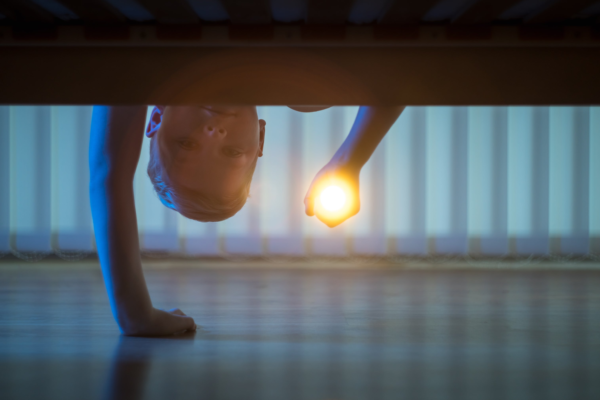
With GPUs, you don’t have that visibility or the flexibility to request, “I want four gigabytes of that GPU, and I only want one gigahertz of that GPU to go with it.” Instead, the most common setup today is all or nothing—you request the whole GPU or none of it. The transparency challenge is that GPUs require an approach to monitoring and understanding usage that’s all their own, because GPUs are specialized and combine aspects of CPU and memory. That challenge is compounded by the fact that a node can have multiple physical GPUs in a system (sometimes up to eight). It’s also possible to add or remove GPUs from systems. That’s something typically seen in on-premises environments, and something you’d not typically see with CPUs. Those dynamics illustrate why gaining GPU visibility requires a fresh approach.
How Kubecost enables GPU monitoring and optimization
Kubecost meets the GPU visibility challenge by understanding which nodes have GPUs and whether those nodes are on a public cloud provider or in an on-premises environment. Kubecost also understands what those nodes cost, and therefore understands proportionally what the GPU costs. That’s true whether a business uses one of the “big three” cloud providers, or self-provides node costs based on its own private cloud configuration.
With those GPU costs in hand, the next step is to look at GPU utilization. Kubecost identifies cost allocation based not only on GPUs requested, but also on GPU usage, in order to recognize idle capacity. Kubecost also scrapes standard metrics, including utilization information, provided by Nvidia software. (We plan to expand to AMD and additional GPU brands.) By combining cost and utilization information, Kubecost can determine GPU efficiency, which is one of the biggest questions in business leaders’ minds as GPUs grow ever more powerful and more expensive.